Maps and Legends / 2023-05-02
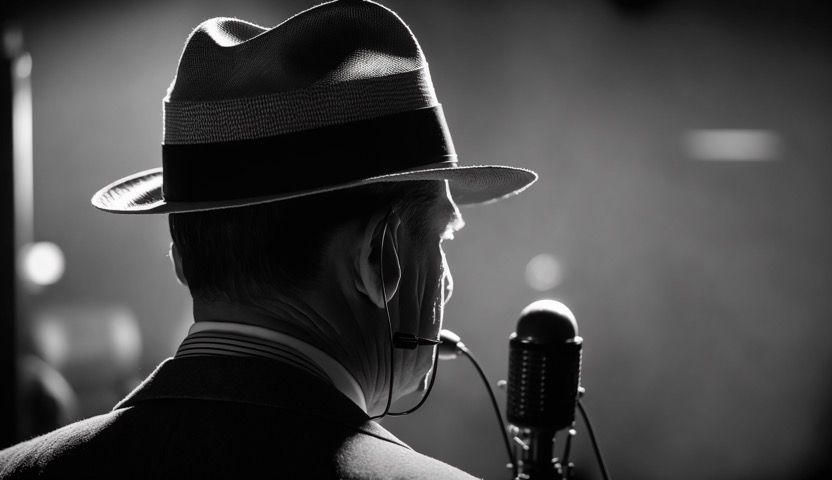
Intro
This newsletter has been fairly deep in the weeds lately, so I thought I’d start by sharing two recent long-but-good intros to core AI topics. The first is “A Very Gentle Introduction” from Mark Riedl, attempting to explain core Large Language Model concepts without lots of technical detail—highly recommended for attorneys. On the flip side, Pam Samuelson of UC Berkeley (one of the world’s top copyright scholars) gives a great intro to the copyright issues around AI. Both are recommended if you’re just getting your feet wet in this space.
Related(?), here’s the shortest possible argument for open(ish) in the ML space, from StabilityAI’s CEO:
Lots of people don't get open source AI is inevitable & required.
— Emad (@EMostaque) April 22, 2023
Regulated & private data is never going to be sent to black boxes with unknown data.
Will be legally required to use open models to turn that regulated data into knowledge.
Folk also want to own their weights.
Events
(All streaming unless otherwise noted)
- I will be moderating a panel on Tuesday May 2 (today!) on AI and open culture with leading attorneys from Creative Commons, Internet Archive, and the Wikimedia Foundation. Should be great! Video will be available afterwards if you miss it.
Values
In this section: what values have helped define open? are we seeing them in ML?
Lowers barriers to technical participation
- HuggingFace is releasing a chat interface aimed at lowering barriers to creation of ChatGPT-like user experiences. The code is Apache-licensed. The current public demo is based on the FB-restricted LLaMA model, but in theory could be ported to other models.
- Related: GPT4All, a locally-installed chat, MIT-licensed UX and Apache-licensed model
- LIT is another “distributed training” initiative, allowing many computers to cooperate to train models. My hunch is that these will be too slow to be competitive in the current generation of AI tools, but it does point to a longer-term future where training may not be so centered on the largest pools of compute.
- Google researchers show substantial performance wins in… Stable Diffusion. Worth noting when researchers end up working on open(ish) models, even when their employer has proprietary equivalents.
Enables broad participation in governance
- CEO of StabilityAI says they’ll have a “broad conversation” on licensing of model weights, after a recent not-open text LLM release. This seems to be consistent with signs from Stability that they are moving away from RAIL, but unclear on what they’re doing next.
Improves public ability to understand (transparency-as-culture)
- “Many eyes makes all bugs shallow” has many limitations, but one of the key ones in the current moment is simply that we often can’t agree on metrics for “bugs”. Here’s a new attempt to quantify gender bias, an important class of LLM bug. The technique relies on a suite of questions that probe gender bias (such as assumptions about the gender of lawyers and secretaries). It finds that GPT-4 is about 3x as likely to answer questions in the stereotyped direction as anti-stereotyped (i.e., 3x as likely to assume that men are lawyers). If we’re really going to improve public understanding of ML, we’re going to need a lot more academic work that establishes quantitative baselines (and conversely, if we don’t have good metrics, we’ll be unable to regulate ML effectively). Related: here’s a public thread poking at some of the same problems, by asking LLMs to self-diagnose their own (biased) grammatical mistakes.
- “51.5% of … sentences are fully supported by citations and … 74.5% of citations support their associated sentence” 35% of sentences are fully supported by citations sounds… pretty good to me? Certainly better than what I get in most history books, much less normal human conversation. But the linked paper finds that generative search tools have that sort of citation level—and argues that it is too low, I assume against a background assumption that every sentence should be cited? I don't know what to make of this—every sentence reliably cited sounds great, but also a much higher expectation than anyone has ever had of anything. Maybe that's good!
Shifts power
- It's long been noted that software monocultures have security problems. New observation: if we end up with an ML monoculture, it will lead to new forms of correlated failure, particularly when many organizations use the same tools to help them make important decisions. Imagine, for example, if many companies all ask OpenAI-based tools to help them make investment decisions based on the probability of a recession. They'll likely all be wrong—in the same, correlated, way.
- CEO of Medium says it is “public knowledge” that AI companies are going to pay for training data. Unclear what he's basing this on, but if he's right, if will substantially concentrate industry power.
Changes
In this section: ML is going to change open—not just how we understand it, but how we practice it.
Changing regulatory landscape
- New lawsuit against LAION in Germany. Details are lacking, but the litigator—a stock photographer—had previously asked LAION to remove his images from the data set, and LAION responded by telling him the claim was copyright abuse and sending him a bill for their legal work(ironically, available to me via ML translation tools). If any of the German lawyers reading this want to weigh in, let me know—happy to share your analysis.
Misc.
- The Wikimedia Foundation legal team has published a preliminary legal analysis of ChatGPT for the Wikimedia community. These "wikilegal" analyses are necessarily high-level and hedged, but still a useful peek into how Wikipedia is looking into this problem.
- The Open Source Initiative’s Stefano Maffuli on “things I learned at Brussels by the Bay”, an AI- and EU-focused set of panels in SF.
- How accounting favors AI over humans, as a result of accounting treatment of spending on "capital" like AI over operation expenses, aka "humans". I’d love a more technical, dispassionate treatment of this—this one is fine, but assumes more knowledge than is ideal.
- Discourse, the “Wordpress of Forums”, is integrating ML. I found the list of ML providers at the end particularly interesting.
Microessays
On commons
I suspect that many (most?) contributors to our various digital commons don’t really think of themselves as members of “commons”. They’d say, more than anything else, that their primary motivation is scratching their own various itches. And yet, given cheap storage and easy collaboration, those itch-scratching instincts have created many excellent commons that others have built on—Wikipedia, Internet Archive, open source, the web as a whole.
But when commons aren't consciously built, they can be unconsciously neglected. My day job has been concerned with that for years, but increasingly I wonder if we're also going to see it in many spaces as a result of ML. These thoughts are still very, very preliminary, but some observations:
- Overwhelming the moderators: Our functioning commons need "gardeners", and (as I've been documenting on this thread) ML is overwhelming those gardeners by helping spammers increase their volume.
- Polluting the commons: I tend to think that the threat of deliberate ML-created misinformation is overhyped sci-fi distraction from real threats, but ML creating bad content that then feeds into other MLs is already here. Wikipedians are familiar with this problem (we call it "citogenesis") but the scope of the coming challenge seems much bigger.
- Shifting creator incentives: If you can get private "good enough" answers instantly from an LLM, why go to the extra effort to participate in a creative community like Stack Overflow, Flickr, or Wikipedia that is slower—but creates a commons as a side-effect? One major Stack Overflow contributor ponders the question here, and Stack Overflow acknowledges and wrestles with the challenges (and possibilities) here.
This is not simply an abstract problem—the LLMs that are creating this problem are in large part trained on these same commons. If Flickr withers because people stop creating useful CC-licensed stock photography, what's the impact not just on us as humans, but on our LLMs?
Other people are starting to think about this in more depth (this is very good) but I suspect it'll become a recurring theme here.
Copyrightability of models
At my recent conference in Sweden, a recurring topic of discussion was the copyrightability of models. I suggested on stage that I was vaguely against copyrightability of models, and someone asked me “why”. Quick sketch of my position:
My claim about the copyrightability of models is fairly limited—I’m not saying that’s the right policy outcome. I'm simply saying that the choices made by the people who do training feel much more like engineering choices, creating a functional thing, and/or sweat of the brow, rather than creative, authorial choices resulting in a work. In significant senses it’s much closer to patents than copyright—make functional choices, run experiments, see what functional outcomes occur. (This is not to say that they should be patentable either, but if I were going to craft a new regime I’d borrow first from patent—particularly the disclosure requirement—before borrowing from copyright.)
The policy question is much harder, I think. I don't love more copyright as a solution, but if there isn't an IP right then model creators will just use SaaS and trade secret. This outcome would be strictly worse for all parties—including the public. We ultimately want these things public so that they can be experimented on, analyzed, etc.; if they’re locked behind trade secret walls that does not do us (collectively) any good.
Closing note
I don't think this newsletter is going anywhere, but it feels like many of the trends I've been discussing are getting more real—expect that, if I have time, we'll start getting more in the weeds about specific licensing decisions and discussions, and more concrete tradeoffs from each of them.
Member discussion