Is this thing on? / 2023-08-20
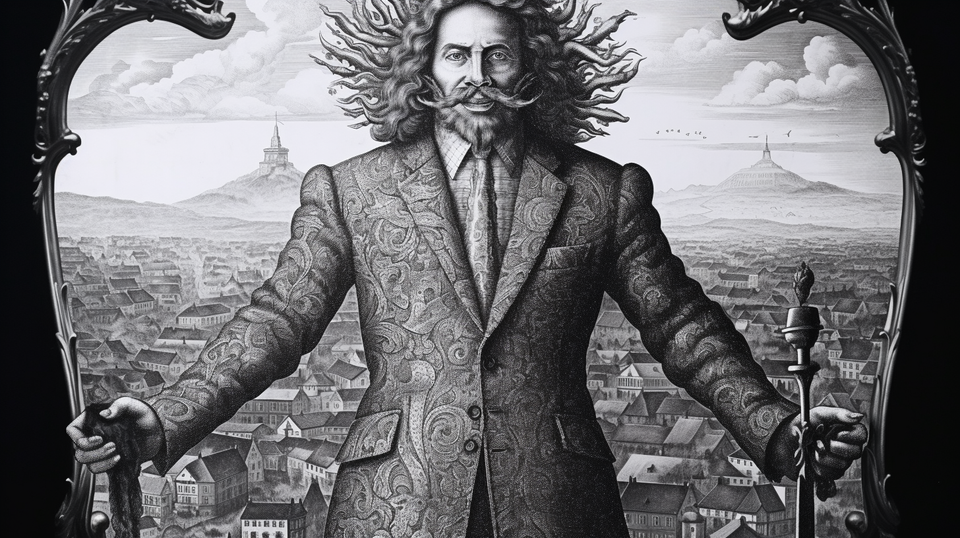
It's been a busy few months of travel, family vacation, and work stuff. So haven't had a lot of time for this side project. (Also a browser upgrade wiped a few dozen tabs that were destined to be "news" in the "newsletter".)
But in the meantime the world—open, ML, and open+ML—have all been moving on. This edition will be mostly catching up on some big-picture stuff, as a result.
Uncertainty
I share Jack Clark's concern in this tweet:
I also think lots of the current debates about AI (especially those relating to intersection of AI safety and AI policy), involves lots of people being extremely confident about what 'should' be done. My general interpretation is it's quite hard to be confident about any of this.
— Jack Clark (@jackclarkSF) August 19, 2023
It’s frustrating not to be able to give honest answers about policy when you genuinely think “we don’t know yet”, and people want you to answer Now Now Now. I hope that has always come through here!
Open and Power
A new paper was published this week on the relationship of open with power. I think the paper is flawed—and very important anyway.
In short, the paper argues that:
- "open", broadly, tends to concentrate, rather than distribute, power;
- "open", specifically in the machine learning case, is technologically/economically extremely difficult; and therefore
- we should be extremely skeptical of claims (such as those from Facebook, about which more below) that "open" machine learning will decentralize or otherwise impact power.
There's a lot to critique in the paper's historical claim of (1) (open concentrates power). Book-length treatises could (and should) be written by historians of tech and antitrust attorneys/economists on that question. I think overall the record is not as grim as the paper makes it out to be, but the paper is absolutely correct to call out that "openness" has been used repeatedly as a centralizing, competitive weapon, and has also in many cases ended up in that situation even when the developers and companies involved genuinely intended otherwise. The paper relies a lot on Android to discuss this, but I would have gone with WebKit, which is open software—with a genuine community history!—but whose ubiquity is being wielded to undermine the open web as we speak. In any case, regardless of where you think the balance falls, open's impact on power is definitely (at best) a grey area, not a clear win for “open”.
Update: Paper co-author Meredith Whitaker clarifies that the paper's claim is intended to be narrower than I've read it as here: "We ... tried to make the point that what is happening (and what happened in the past) is an instrumentation of open source by corporations in service of increasing power, but that such isn't an inherent quality of open source itself." I'm pretty agreed on that claim, and think it's worth a lot more exploration, since growing corporate comfort with open makes this easier—a facet of "we won, now what" that we don't talk about enough.
Similarly, I think (2) (open is impossible in ML) is an open question and will be for a while, as we explore both the economic and technological space around ML. ML is certainly expensive and difficult now, and we should take that challenge seriously when analyzing policy. But as I've been writing about here for some time, there are plenty of reasons to believe that (as a technical matter) ML can get cheaper and easier (eg from June), and that (as an economic matter) lots of parties will invest in making it cheaper and more accessible. Again, though, where this falls on the spectrum is grey—open may get there, but it certainly may end up in a local maxima where hardware, data, and techniques don’t really allow an “open” that generates the positive social outcomes we tend to associate with openness.
It’s exactly because of these grey areas that I whole-heartedly support the authors' conclusion: "open" is not a magic bullet to the heart of power. We should all scream from the mountaintops that "open" can centralize power, either unintentionally or as an explicit strategic goal. They are also correct that we need to be particularly concerned about the relationship of open and power at a time when the means of technological production are firmly centralized. In other words: we can't just say "open is good"—at least along the dimension of societal and industrial power, open can and will be used and abused by those who seek to control the rest of us.
So what does this mean for the existing open source software community? I think that the open community must acknowledge this challenge, backing off strong claims that open is always "democratizing". And it must consciously, deliberately fight any use of "open" as a rhetorical weapon that gives giants like Facebook (and OpenAI) a way to avoid scrutiny from society, labor, and government. (The paper is quite strong on how OpenAI, in particular, abuses open in this way—but as I'll discuss below it is just as problematic from Facebook.) It should also look to build tools, institutions, and social movements that tilt the playing field towards a healthy openness, rather than a centralized one.
This attitudinal change won't be easy for traditional open source. There's decent historical reasons why open advocates argue that open source decentralizes power: when "open source" was coined, the pre-eminent cultural concern about computing ethics was the power of Microsoft. At the time, the belief that open could challenge that centralized power was not only widespread, it was correct! Mozilla, Linux, and the BSD-based MacOS/iOS, were all critical in successfully challenging an otherwise very durable set of interlocking monopolies.
The challenge for open is to recognize and claim that important history without resting on two-decade-old laurels. We still haven't really come to grips with how Linux helped re-centralize power with the three major cloud providers. And we all badly want to believe it'll have similar impact in ML, but the evidence for that is thin at best. So this change is important—but it isn't going to be easy.
So, despite my concerns, I strongly recommend that everyone in open—not just in open ML—read this paper. You may not agree with it, either in the specific or on balance, but I think it provokes in an important way and I look forward to strong critical responses to it.
Facebook and Open
I've been doing other things during most of the kerfuffle around whether or not LLaMA2 is meaningfully "open source", as Facebook and Zuckerberg have repeatedly claimed. (It clearly isn’t.) But the issue will remain going forward as long as Facebook insists on using "open source" to describe LLaMA2.
A few observations:
- power matters: For a variety of reasons, I've argued for a long time that we need to be generous when people misunderstand "open", and tolerant of near-open approaches. But we still have to look at who is saying and doing it. It's one thing when young students trying to make the world a better place get confused, and another when, well, noted PHP enthusiast Mark Zuckerberg does it. That’s true even if you don’t think the motivation is openwashing—regardless of the motivation, the volume and power with which the message is projected increases the damage.
- definition matters: Related to the previous point, it's one thing when a handful of activists challenge the definition of open source. It's another when a huge PR blitz (which is what this was, with articles in every major tech news outlet) mis-represents open source. The entire software industry relies on a shared, agreed-upon definition of open source to reduce developer and legal costs. If Facebook breaks that shared understanding by misrepresenting “open source” to the media and developer public, it's a big problem for all of us—including Facebook. Stephen O'Grady goes into this in a lot more detail. If you want to go even deeper, read up on open source as a "Schelling point".
- this isn't focused: a lot of the most interesting attempts to do near-open or open(ish) tend to push very hard on one particular assumption of the current definition of open source, while embracing others. For example, the Ethical Source folks actively rejects the "no restrictions on use" plank of the Open Source Definition but embrace other community-enhancing parts of open. Facebook's terms (and approach) break the Open Source Definition in not just one, but several ways—they discriminate against specific users; they discriminate against specific uses; they do not provide even the slightest hint of the ability to rebuild the project's core artifact; and they produce uncertainty (reducing investment and innovation). Its only real benefit is low cost (and mayyyybe certain types of amateur innovation) out of the many axes of open.
- this isn't articulate: I don't agree with Hashicorp's recent change away from open source, but at least they've got a fairly clear explanation of why. Facebook has made very little attempt to explain why they chose the restrictions they did, which makes PR blitz even worse. This could have been an intriguing opportunity for a real discussion of where open should go, if Facebook could have articulated why they're doing this. But they didn't—so instead it's left to the open source community to point out the confusion.
In short, if Facebook wants to have a real discussion about what open(ish) should look like in machine learning, I for one would welcome that—it's far from clear that "pure" open makes sense in this space. But the community should (and mostly has!) go hard after them for, instead, simply bigfooting 25 years of industry investment and practice.
Stopping open?
At GenLaw, the most interesting discussion I had was with an attendee who made a strong argument explicitly against open in the AI space. This was not just as a technical matter (i.e., that it won't be possible) but that as a legal matter it shouldn't be allowed by a competent government.
Many of their arguments were strong, and as I noted in my last essay, I'm open to being persuaded that open(ish) ML will either be technically difficult, or a net-negative for society. (I'll hopefully essay in more depth on that soon, to help me work through my complicated thoughts on that.)
But as we sparred, I kept coming back to a really challenging problem: if you don't think open(ish) ML should be allowed by The State, and all the technical trends point towards it becoming easier (albeit not necessarily easy), what's the mechanism by which The State will enforce such a constraint?
I think a lot of tech civil libertarians who are legitimately very concerned about privacy, bias, and other issues with ML, and particularly with open ML, have not yet grappled with the fact that the leading proposals to curb the impacts of distributed ML are extremely dystopian. This is not me exaggerating; here's a major think tank in the space (an original funder of OpenAI) suggesting that all "cutting edge" chips (undefined, but recall that every new Apple device can do reasonably fast inference) should include the option for remote government shutdown (point #2). This makes Clipper chip look tame! And "cutting edge" (like every other technique for seeing like a state) is not going to stay limited; once an infrastructure for such shutdown is built, it will be in every mass-market computing device within a few years. Perhaps that intrusion is, on balance, worthwhile, but if you're making the case for eliminating open source ML, that's the case you have to make.
There's a lot of good policy recommendations in that post too, most of which I agree should apply to open AI models (however one defines open). (Lots of good stuff in here too, stopping short of anything quite so draconian.) But at the end of the day, given that many places (China, Singapore, and the UAE, just to name a few) are creating and releasing open models, the only way to prohibit open is going to be extremely draconian control of general-purpose computing.
Lots more miscellany
Miscellaneous news from the past couple months:
- One of the challenges of iterative improvement of models is that training has been one way—data, once trained into the model, is very hard to remove. Research is pushing on that, though—this new paper suggests a technique to remove entire concepts from models. While the most obvious impacts of this are regulatory, it’s also good for iterative development—which is critical to open. On the flip side, a paper at GenLaw suggested that such removals could make other related concepts even more discoverable. There’s still a lot of work to be done here :/
- open(ish) ML is starting to come to climate, in the form of a model from NASA and IBM. NASA is saying that this will be open consistent with the White House’s policy on open science, but I’ve been unable to find details. (Unfortunately, there is also new work on water usage of ML models, and it isn’t pretty.)
- There’s been a lot of tests of attack vectors on ML models lately, but this is the first systematic approach that has come across my radar. It’d be interesting to run through this for some of the most popular models (open and otherwise) and see any systematic patterns.
- While LLMs continue to get all the headlines, non-LLM machine learning continues to make really interesting impact. Over the summer, Google’s Deep Mind enhanced basic sorting algorithms to discover a way to improve the efficiency of the widely-used LLVM libc++ sorting library—making literally millions of programs faster. I expect that even if “open” never really has impact in LLMs (for regulatory or other reasons) we’ll continue to see stories like this out of different ML approaches, and they’ll increasingly rely on open components and techniques.
- I continue to wonder about how ML will force us to think about “commons” in a deliberate way. StackOverflow may already be feeling the brunt, as people move from collaborative Q&A to 1:1 interactions with an LLM. Wikimedia is also being proactive in this area.
- This is a really good, short post on the respective intellectual arguments for the two sides of the “schism” in machine learning ethics—in short, “there isn’t a true debate”, at least in the academic literature.
- Say what you will about LLaMA2 (and I said a lot above) I can run it on my Mac thanks to a lot of interesting open source work.
Member discussion