Ghosts in the machine / 2023-10-19
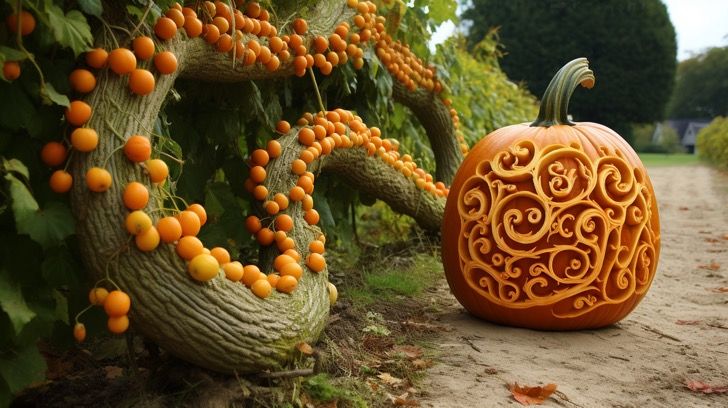
Welcome new subscribers!
Welcome new subscribers! You might start with my essays on why machine learning might be as big as the printing press (and how we underrate the impact of the printing press!) and on why I am not (yet) a stickler for precise usage of “open” in this space.
Recordings: past
- The Open Source Initiative has published videos of its recent deep dive series, including me on the RAIL licenses (where, despite what I said above, I spent a lot of time being a stickler about open).
Events and recordings: future
- Linux Foundation is doing an open “GenAI and ML” summit in San Jose Dec. 12-13. I’ll probably be there, please say hi if you will be as well!
- Mozilla is doing an entire podcast series on AI.
Values
In this section: what values have helped define open? are we seeing them in ML?
WTF is open anyway?
I’m not the only one looking at the possible facets that get bundled into “what is open anyway”—because lots of things we assumed came together in the past may no longer be that way, unless we do it consciously. Here’s a few recent lists of those facets:
- Here’s one spectrum, though not sure I agree with the conclusions (and not sure it’s even a spectrum).
- Here's a wildly detailed chart that not only provides a list of facets of openness, but analyzes a pile of actually-existing models by that list.
Lowers barriers to technical participation
This giant list of open LLMs is a good starting place for looking at the actual shape of open(ish)-in-the-wild, with the changelog showing how the space is growing. But I can’t stress enough that this list is based on self-reported “open” and so may not actually give the standard-OSI-open rights.
Enables broad participation in governance
More about this below, but it’s interesting to call out this one slide from a Wikimania talk on ML at Wikipedia—about how they’re thinking about model cards as part of community governance of models.
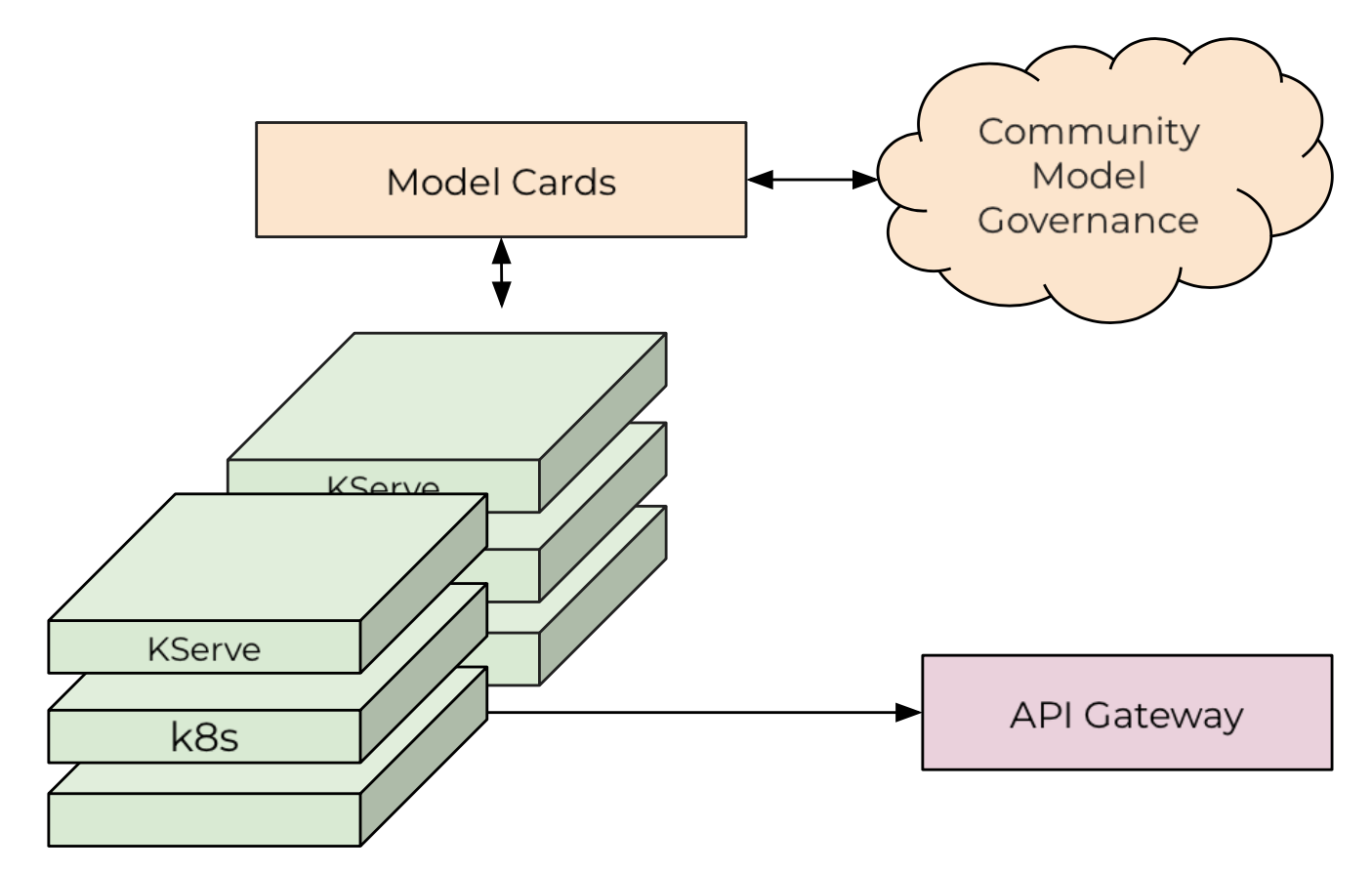
Chris Albon of WMF has talked more about cards-as-governance as well; I’m super-curious to see how this evolves.
Improves public ability to understand (transparency-as-culture)
This section has often been empty/missing in these newsletters, perhaps indicative of the lack of focus on this area in the broader community?
But this paper is an interesting example of how ML’s different architecture from makes reverse-engineering—and so accountability/transparency—possible. It reverse-engineers CLIP (a key part of image training models) to help understand and improve it. I expect we’ll see more of this (though more next week on what kind of research gets prioritized, or not, and what that says about the prospects of open.)
Shifts power
- In “not shifting power”, here’s HuggingFace announcing that they’re taking piles of money from a lot of very familiar names in VC. There’s nothing wrong with this, but it’s an important part of understanding HF’s relationship (in the long run) to power.
- This “gpu-rich/gpu-poor” analysis has spawned memes and is an important complement to the paper on “power” I discussed in the last newsletter. (It’s a subscription link but the important part is above the paywall fold.)
- This AI Now Institute piece explaining computer in ML is very long but good. Its short-but-sweet policy section” focuses on antitrust as a key concern—something which I think has been neglected because of the overfocus on copyright/IP law. (Here’s another piece making the same, pro-antitrust, argument.)
- In maybe-sort-of-shifting-power, the Linux Foundation is getting into open data in a big way. LF is perhaps the epicenter of the “open shifts power, but to whom” question posed by Whittaker, Widder, et al in the last newsletter.
Techniques
In this section: open software defined, and was defined by, new techniques in software development. What parallels are happening in ML?
Deep collaboration
- Moderation is part of how communities grow, so here’s Hugging Face announcing their new community policy. It emphasizes consent—which is somewhat weird in a scrape-first, ask-questions-later industry. Consent for me but not for thee?
- Nathan Lambert has been on fire lately; here he is from earlier in the summer on the path-dependency of different LLM talent pools, which I think is going to be very interesting to watch—just as open source software really appealed to hard-core systems guys (using guys on purpose here!) and failed to attract UX researchers, it seems certain that the shape of the humans who work on this will affect the shape out of the outputs. More on this next week, via a paper that hit while I was writing this…
Model improvement
- Not model improvement per se, but here’s a deep dive on the lightweight, open C++ implementation of LLAMA, llama.cpp, explaining how you can get great performance on smaller devices this way—but also, critically, noting that the technique is not necessarily generally applicable—so we should shouldn’t expect that this will work on all models.
- Despite those caveats, it did work on LLaMA2, almost immediately.
Transparency-as-technique
Open access does not automatically create transparency—you need to be able to not just access, but also evaluate. Some links on how the space is maturing here:
- Data and Society has launched a new “Methods Lab”, focused on evaluating ML. I look forward to seeing what comes out of that.
- Stanford has created a scorecard of models on (draft) EU AI Act compliance. TLDR, compliance is woeful. And despite that I’ve heard from Europeans that Brussels found even the attempt in this scorecard somewhat laughably incomplete.
- Here’s an interesting attempt at benchmarking “factuality”.
Availability
- Wikipedia has a good presentation on how they’re using Machine Learning. Interestingly for the availability point, for translation, they’re moving away from third-party hosted models and towards self-hosted models—something that would have been difficult to picture a few years ago. But they also note that their new LLM-based spam-prevention tooling is “heavy on computation resources”.
- Allen Institute drops a truckload of training data under an open(ish) “medium-risk” license. Good news: the documentation is, as best as I can tell, best-in-class. Bad news: there’s a lot of it and I still haven’t fully digested it yet.
- Meta steamrolls on with new translation model and new (very powerful?) code model.
Joys
In this section: open is at its best when it is joyful. ML has both joy, and darker counter-currents—let’s discuss them.
Humane
- One of my favorite areas for open content is so-called “Galleries, Libraries, and Museums”—GLAM. Here’s a guide from Hugging Face on using ML for GLAM.
Radical
- I’ve been interested in technology as a tool for democratic deliberation since the late 1990s, and here’s an attempt at using “LLMs for Scalable Deliberation”. I don’t think you can reason your way out of the deep bias problems here (that’s what torpedoed my writing about it in 2000-2001), but it’s still an interesting thought experiment.
- Using LLMs to generate SQL is the kind of thing that might be very empowering for a lot of people who want to query data but don’t know SQL. Or (given that state of the art accuracy is only 80%) it might drive a lot of frustration. In either case, note that this is being done with open(ish) foundation models; I suspect Snowflake would otherwise not have the capacity to do this.
- Today in impacting the “real world”: using models to reduce the climate impact of plane flights.
Changes
In this section: ML is going to change open—not just how we understand it, but how we practice it.
Creating new things
I’ve enjoyed toying with this new, focused-on-a-fun-frontend-to-customization ML tool from a team of Mozilla vets. Recommend playing with it. The core insight: “view and edit prompt” now has a lot of vibes similar to “view source” in the early days of the web.
Ethically-focused practitioners
- I tend to think that literally doing street protests against open-ish ML is a stunt rather than a serious statement, but it’s at least an effort to ethically engage—the industry could use more of that. (see also)
- “Open” is a cross-disciplinary concept now, and so it’s very interesting to see survey data on open-related attitudes across time in science. TLDR: attitudes towards sharing are improving, but not in a consistent way across industries or regions.
Changing regulatory landscape
- This is a good summary of the challenges of enforcement in the EU AI Act. As I mentioned in the last newsletter, enforcement is going to be a real challenge—especially as this stuff grows.
- related: will genuine, community-driven open get a seat at the table? Here is stability.ai’s submission in support of “grassroots innovation” through open (pdf, twitter thread)
- NTIA solicited comments on AI Accountability. Some key comments: Hugging Face, Anthropic, Princeton-Stanford.
- For the lawyers, a long-but-interesting paper on the perils of “custom” IP regimes. TLDR: what happens when an industry thinks it should get a custom type of IP? It ends up being pretty useless, typically. Relevant here since there will be at least some temptations to create “custom” rules for AI-built IP.
- So far, San Francisco and California are completely failing to have a healthy conversation about governing self-driving cars, with a seeming binary of either completely ungoverned unresponsiveness, or holding AVs to an impossibly high standard while dozens (in SF) and thousands (in California) die each year from human-driven cars. This is in some way the highest-stakes, and most-advanced, discussion about AI regulation—and it doesn’t bode well, I fear.
Misc.
- Benedict Evans has a very good point: large-scale change, even when clearly beneficial, can be very slow; that’s not just a truism, there’s a lot of data to back that up. This is the strongest counterpoint, IMHO, to the “this is printing-press-level change”.
- Algorithmic justice issues are not new; here’s a very academic paper on how water optimization algorithms encoded injustice as far back as the 1950s.
- Here’s economic research on how technology increases state capacity, which increases the state’s penetration of people’s lives. Here’s unrelated news about ChatGPT being used to ban books, because the overworked-underfunded librarians could either use ChatGPT or shut everything down.
Member discussion