My commons roller coaster / 2024-10-10
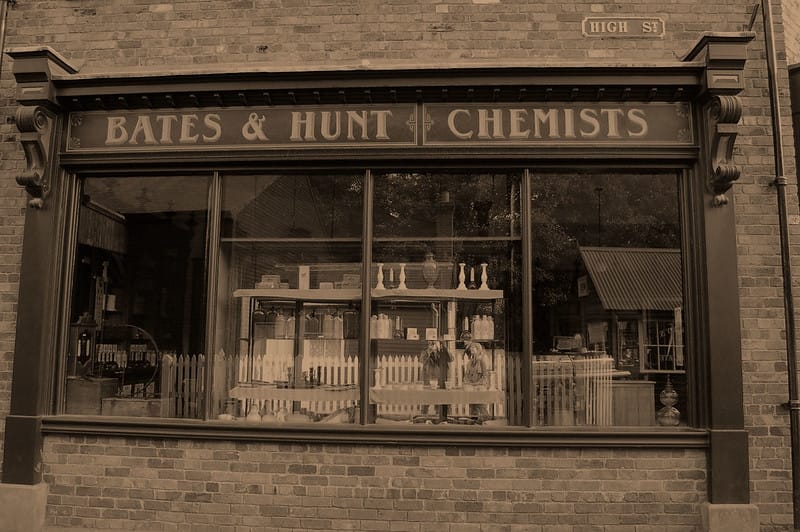
This week an open data set won the Nobel Prize in chemistry... and I’m not sure I even want to talk about it.
(Also, hi, I’m back! At least for today.)
A Nobel for a commons
Let’s take a step back: a few months ago I mentioned DeepMind’s AlphaFold as an interesting practical implementation of modern AI—using existing protein data as the core training data set for a model that can now predict the universe of all proteins known to humanity.
Critically, that existing protein database is a spectacular example of modern scientific open data and science: a collaboration across decades, involving scientists from all over the globe, with support from governments and institutions, creating a dataset truly licensed to the entire public under the Creative Commons “Zero” license. AlphaFold used that very public data as the core of their work.
This week, the leaders of the AlphaFold team were awarded the Nobel Prize in Chemistry for that work. To quote the announcement about the impact of the work:
That we can now so easily visualise the structure of these small molecular machines is mind boggling; it allows us to better understand how life functions, including why some diseases develop, how antibiotic resistance occurs or why some microbes can decompose plastic.
So to say an open data set won a Nobel is a little bit of an exaggeration… but it’s not an exaggeration to say that without the open data set the Nobel-winning, world-altering work would not have happened.
And so I should be thrilled: my life’s work, of building common resources that can be used to move humanity forward—validated! Right? TLDR for the rest of this email: I’m in mourning for other open data, which makes it hard to celebrate this win.
Obituaries(?) for a commons
A few months ago I was an advisor on the Data Provenance Initiative’s paper on changes in Terms of Service over time—subtitled dramatically, but essentially. correctly, “the rapid decline of the AI data commons”.
There’s a lot going on in the paper, but the short version I want to highlight goes like this:
- identify what the top public websites used in training data sets are
- grab the legal terms for those websites via Internet Archive’s Wayback Machine
- analyze the legal terms to see if they’re getting more restrictive over time
In short, websites absolutely are rapidly and firmly changing from “sure, use this“ to explicitly requiring a variety of different permissions:
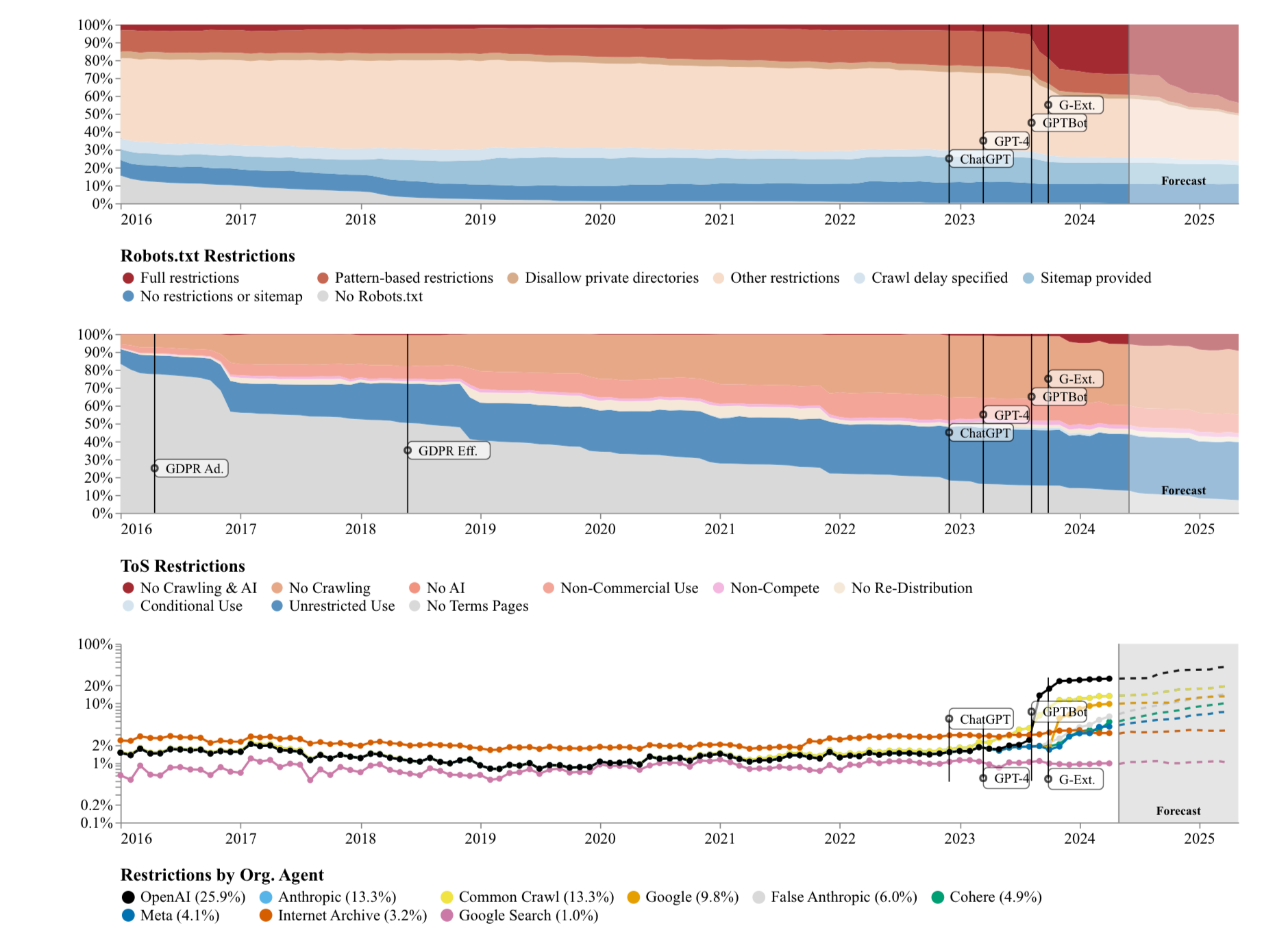
Shortly after the paper was published, Cloudflare, which claims to power something like 30% of the web, announced that they’re going to make it easy for their customers to opt-out of AI training, and indeed all crawling for any purpose.
Whether or not the web was a “commons” is complicated, so I don’t want to push that label too hard. (As just one example, there was not much boundary setting—one of Ostrom’s signals of a traditional common pool resource.) But whether or not it was, strictly speaking, a “commons”, it was inarguably the greatest repository of knowledge the world had ever seen. Among other reasons, this was in large part because the combination of fair use and technical accessibility had rendered it searchable. That accessibility enabled a lot of good things too—everything from language frequency analysis to the Wayback Machine, one of the great archives of human history.
But in any case it’s clear that those labels, if they ever applied, very much merit the past tense. Search is broken; paywalls are rising; and our collective ability to learn from this is declining. It’s a little much to say that this paper is like satellite photos of the Amazon burning... but it really does feel like a norm, and a resource, are being destroyed very quickly, and right before our eyes.
Perhaps that’s for the best—I really am open to the idea that this particular village needs to be destroyed to save the villagers—but nevertheless it triggers in me a sense of mourning; a window that is passing.
The troubled present of digital commons
To be clear, this is not just about my vibes (though that’s a lot of it!) Some contradictory things I’m trying to hold in my head while processing these emotions:
- Common resources continue creating great value for humanity. Hooray! Yay us! We can create new, cool, fun things together and have unexpected, amazing, powerful serendipity happen.
- By finding previously undiscoverable patterns in our knowledge commons, machine learning is creating new knowledge. This is not perfect, by any stretch, and has many implications—but nevertheless, it’s real. We’re able to use machine learning for real, concrete things, and that’s going to advance society.
- Common resources are under threat. I’m a firm believer that much of the best creation is done for the sheer joy of it, but it’s absolutely still bad for creativity if creative people feel surprised and exploited, and their few outlets for compensation are even more squeezed.
- Not all “commoners” understood all the implications of their gifts. This cuts two ways—the ultimate conclusion of the protein data story is a very happy one that could not have been predicted when the data set was created, but the average website is probably not thrilled that their data is being used to create slop that competes with them in Google search results.
- The “commons” label can be exploited. There’s real differences between digital commons and IRL commons, so not all of Ostrom’s concerns apply (or apply differently). But ultimately the Ostromian school is right that a common resource inevitably involves humans in relationship to each other, not just a pile of resources (or worse, “content”). Because of that, we should be careful about applying the label of “commons” to digital data collections—the data may be in many senses a commons, but if the humans who created that commons aren’t on board (or are just surprised!) it will quickly be a challenge.
The troubled discourse around digital commons
All that said, I’ve been slow to write about this (and other things) not just because the topic is complicated. It’s also because it’s hard to have fun discussing it. Arguments are not being sharpened; opinions are not being shaped. Instead, in too many cases, the battle lines have been drawn; the arguments aren’t being improved or revised, they’re just being wielded.
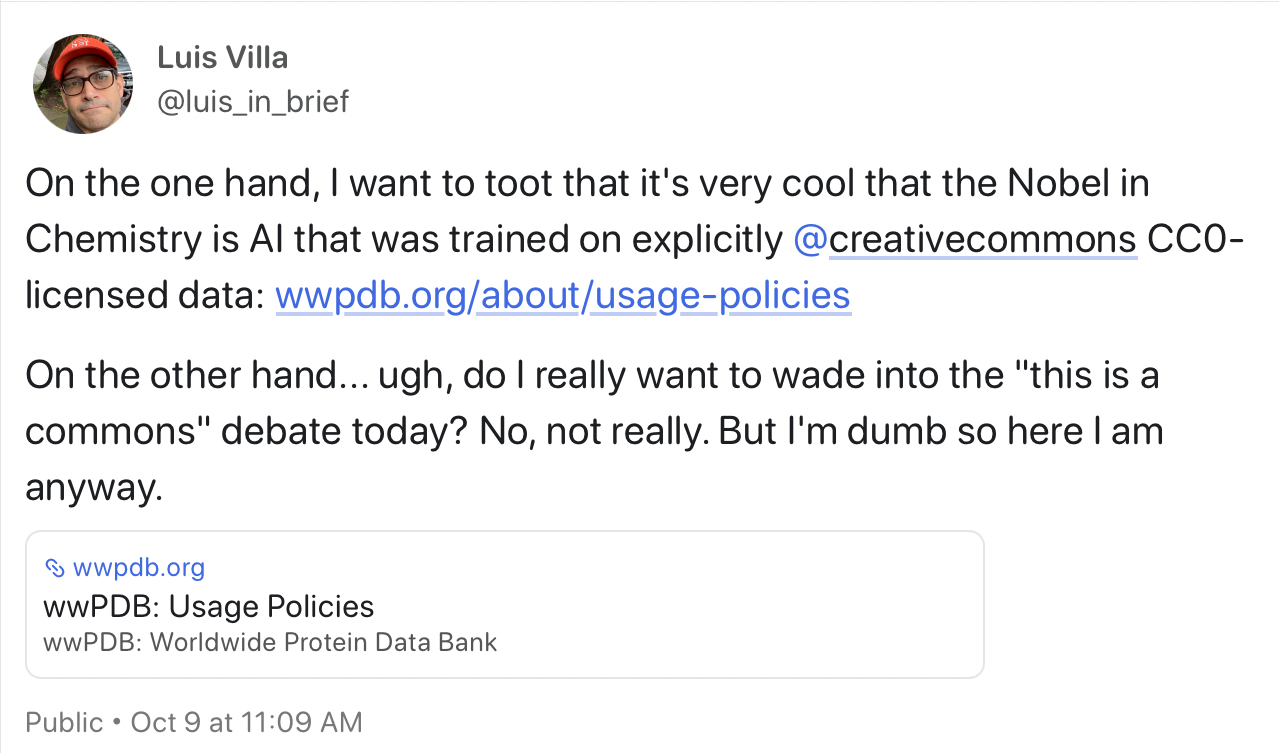
And so writing online around the digital commons, and particularly its use in machine learning, now requires a lot of emotional labor for me. Every statement has to be heavily hedged and caveated; if you don’t signal exactly the right concerns you’re going to get jumped. It feels like there is no way to learn in public.
And to be clear, I’m part of the problem: just yesterday, in response to an old friend’s substantively important claim that there are no important open-data-trained machine learning models, I couldn’t help but snark something to the extent “except for the one that just won a Nobel”. I was correct! (And I think there will be more!) And it’s substantively important too, because if your belief is that open data won’t yield significant models, you’ll come to incorrect policy (and ethics) decisions. But nevertheless... it didn’t feel great to join in that fight.
So about the future?
And so we’re back to the same place I started this newsletter: fierce battles over the smallest possible deltas of opinion, in a field with very high stakes.
For the commons, my core take is a very mundane one: it’s too early to tell, and too early to write anything into stone. If someone tells you that they’re sure, for example, how the EU’s Text and Data Mining exception applies to open source requirements, just wait a month and they might be quite wrong. Some takes might take longer to disprove; I’m now pretty sure my earliest takes on the applicability of Google Book Search to LLM training data were wrong, but we probably won’t know the “right” answer for years—if at all! In the meantime, we’re definitely going to see restrictions that make less data available for training, and we’re definitely also going to see technical work to reduce the amount of data necessary for good performance. Which will win… who knows?
For me personally, I admit I have little appetite to jump into that right now. The highs (a Nobel!) are high, but the lows are very low—considering carefully how to fight old friends, sometimes in public. So expect quiet from me for a while longer while I work out how that works for me going forward.
Member discussion